
And, a number of recent works have shown that this information can be used to build richer individual user models and to discover additional behavioral patterns that can be leveraged in the recommendation process. In many application domains, however, multiple user-item interactions of different types can be recorded over time.
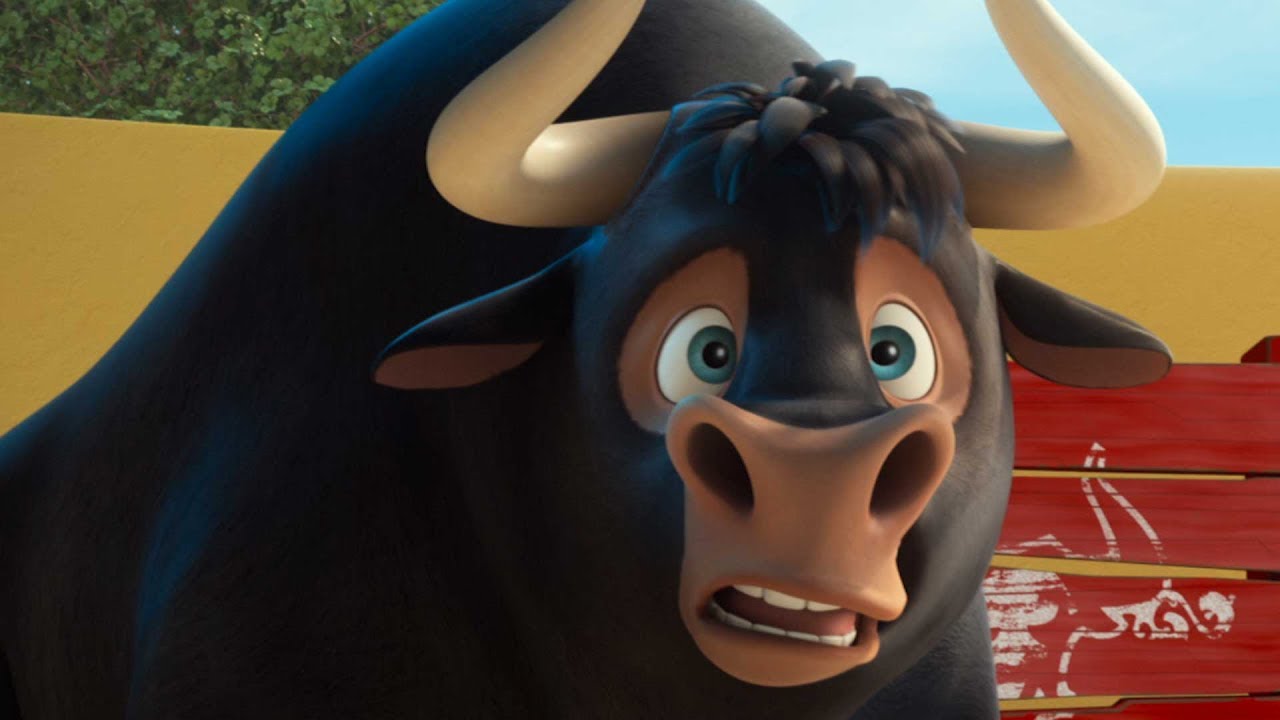
Academic research in the field is historically often based on the matrix completion problem formulation, where for each user-item-pair only one interaction (e.g., a rating) is considered. Recommender systems are one of the most successful applications of data mining and machine-learning technology in practice. Our results therefore suggest that there is substantial room for improvement regarding the development of more sophisticated session-based recommendation algorithms.

Our experiments reveal that algorithms of this latter class, despite their sometimes almost trivial nature, often perform equally well or significantly better than today's more complex approaches based on deep neural networks. Our comparison includes the most recent approaches based on recurrent neural networks like GRU4REC, factorized Markov model approaches such as FISM or Fossil, as well as more simple methods based, e.g., on nearest neighbor schemes. In this work, we present the results of an in-depth performance comparison of a number of such algorithms, using a variety of datasets and evaluation measures. Given the high practical relevance of the problem, an increased interest in this problem can be observed in recent years, leading to a number of proposals for session-based recommendation algorithms that typically aim to predict the user's immediate next actions. In many real-world applications, however, such long-term profiles often do not exist and recommendations therefore have to be made solely based on the observed behavior of a user during an ongoing session. Most academic research is concerned with approaches that personalize the recommendations according to long-term user profiles. Recommender systems help users find relevant items of interest, for example on e-commerce or media streaming sites. Based on the classic 1936 children's book by Munro Leaf." 'Ferdinand' is currently available to rent, purchase, or stream via subscription on Apple iTunes, DIRECTV, Microsoft Store, Redbox, Google Play Movies, Amazon Video, AMC on Demand, Vudu, Spectrum On Demand, fuboTV, FXNow, YouTube, and Disney Plus. As Ferdinand grows big and strong, his temperament remains mellow, but one day five men come to choose the "biggest, fastest, roughest bull" for the bullfights in Madrid and Ferdinand is mistakenly chosen.

Here's the plot: "Ferdinand, a little bull, prefers sitting quietly under a cork tree just smelling the flowers versus jumping around, snorting, and butting heads with other bulls. You probably already know what the movie's about, but just in case.
#Ferdinand nowplaying movie
Released December 15th, 2017, 'Ferdinand' stars John Cena, Kate McKinnon, Anthony Anderson, Bobby Cannavale The PG movie has a runtime of about 1 hr 48 min, and received a user score of 73 (out of 100) on TMDb, which collated reviews from 2,404 well-known users. Now, before we get into all the details of how you can watch 'Ferdinand' right now, here are some specifics about the 20th Century Fox, Davis Entertainment, Blue Sky Studios, 20th Century Fox Animation family flick. Below, you'll find a number of top-tier streaming and cable services - including rental, purchase, and subscription alternatives - along with the availability of 'Ferdinand' on each platform when they are available. Want to behold the glory that is ' Ferdinand' on your TV, phone, or tablet? Finding a streaming service to buy, rent, download, or view the Carlos Saldanha-directed movie via subscription can be a challenge, so we here at Moviefone want to do right by you.
